Monte Carlo#
The Monte Carlo method is a statistical technique that involves running multiple simulations with randomly sampled input parameters to estimate the distribution of the output variables.
In Africa, navigating decision-making amidst rapid demand growth and substantial infrastructure changes poses a significant challenge. To tackle this uncertainty, the Monte Carlo method can be employed to construct a stochastic interpretation of deterministic model scenarios. This approach enhances the ability to account for variability and assess potential outcomes, providing a more robust foundation for informed decision-making.
To use the Monte-Carlo method in PyPSA-Earth, you have to activate the
monte_carlo
option in the configuration file (config.yaml
),
set add_to_snakefile
to true in the monte_carlo section. This will
enable the monte-carlo method.
There are a few additional options that needs to be set in the monte_carlo configuration options.
Set options
#
samples
: The number of samples to be used in the monte-carlo simulation.samppling_strategy
: The method used to sample the input parameters. Either ofpydoe2
,chaospy
, orscipy
.seed
: The seed for the random number generator. It is useful to set the seed to a fixed value to ensure reproducibility of the results.
Set uncertainties
#
The uncertainties
section in the configuration file is used to specify the
parameters to be sampled in the monte-carlo simulation. The uncertainties
section is a dictionary with the keys being the pypsa object value
to be
sampled and the values split into type
and args
of which type
is used to
select the distribution and args
used to specify the parameters of the selected
distribution type.
The following is an example of the uncertainties section in the configuration file:
uncertainties:
loads_t.p_set:
type: uniform
args: [0, 1]
generators_t.p_max_pu.loc[:, n.generators.carrier == "onwind"]:
type: lognormal
args: [1.5]
generators_t.p_max_pu.loc[:, n.generators.carrier == "solar"]:
type: beta
args: [0.5, 2]
Note
To understand the different distribution types and their parameters, check Scipy Documentation
Tip
To create reallistic uncertainties, it is important to pay attention to
the distribution that is being applied to each pypsa object parameter
.
Workflow#
To perform a dry-run of the monte-carlo simulation after setting the config options, use the following command:
.../pypsa-earth % snakemake -j 1 solve_all_networks_monte -n
To create a DAG of the monte-carlo simulation workflow, use the following command:
.../pypsa-earth % snakemake -j 1 solve_all_networks_monte --dag | dot -Tpng > monte_carlo_workflow.png
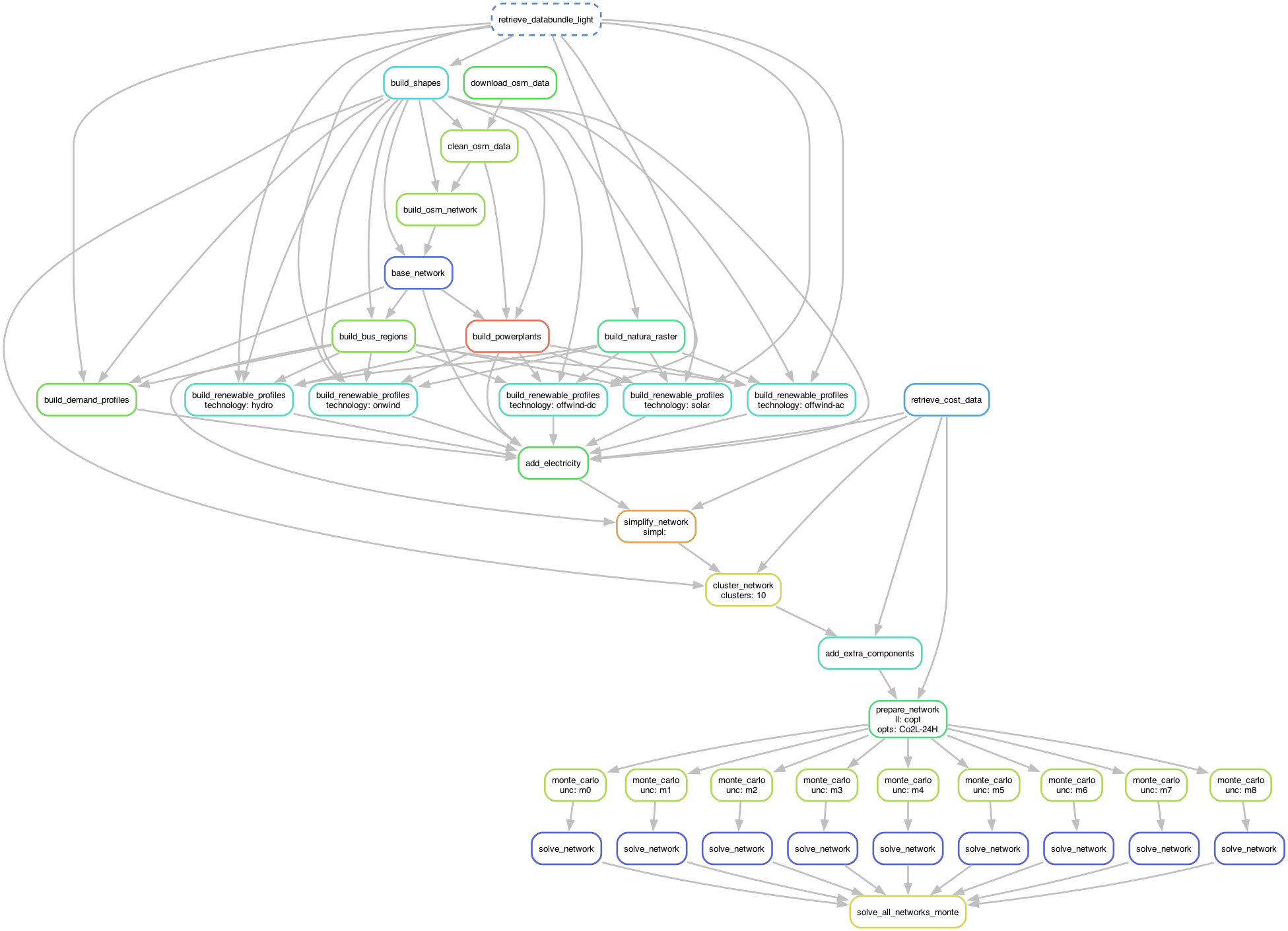
The monte-carlo simulation can be run using the following rule:
.../pypsa-earth % snakemake -j 1 solve_all_networks_monte
Note
Increasing the number of cores can make the process run faster. The numbers of cores can be increased by
setting the -j
option to the desired number of cores.